LC Labs is an experimental division within the Library of Congress spearheading projects at the intersection of people and technology. With a number of exciting explorations in both crowdsourcing and machine learning (ML), LC Labs is playing a key role in growing awareness in libraries, archives, and museum communities around the challenges involved in realizing the potential of crowdsourcing and ML approaches.
LC Labs incubated crowdsourcing projects like By The People that have leveraged the power of volunteers to expand full-text search of historic textual materials through online transcription. Recent LC Labs machine learning experiments showed how models can be trained to create new access points to digitized collections—the Newspaper Navigator project, for example, automatically classified graphical content like advertisements, cartoons, and photographs in historic newspapers to encourage alternative ways of discovering, interpreting, and discovering this content.
The Challenge
These experiments, however, have shown that crowdsourcing efforts may not be enough to efficiently approach data enrichment tasks at scale. And while ML models can scale, they usually need significant amounts of training data to achieve acceptable measures of accuracy.
LC Labs was interested in exploring how they could effectively and responsibly integrate the best of both approaches to create a “human-in-the-loop” process where humans create training data through crowdsourcing for ML models and verify the accuracy of the outputs to improve ML processes.
The Solution
To begin exploring potential approaches for humans-in-the-loop projects at the Library, Labs selected AVP to develop:
- a crowdsourcing and machine learning prototype,
- an interface for presenting the data outputs of human-in-the-loop processes to users, and
- user studies designed to better understand public users’ sentiment towards the use of machine learning processes to enrich collection access at LC.
The Library aims to build lifelong and meaningful connections with users in a responsible way that protects user privacy and safety, so AVP applied a user-centered approach to help LC Labs develop a framework for designing human-in-the-loop data enrichment projects that are engaging, ethical, and useful.
AVP’s crowdsourcing, UX, and machine learning experts collaborated with a core project team at LC to select an appropriate collection for the experiment, design prototype crowdsourcing tasks and ML processes, mock up a hypothetical presentation interface to the data generated from these processes, and conduct user testing. Over the course of the experiment, the project team applied tools such as brainstorming workshops, user stories and persona development, usability testing, prototyping, and wireframing to help the Library better understand how to build a successful and ethical human-in-the-loop project.
Using the Library’s U.S. Telephone Directories Collection as a test case, AVP repurposed existing open-source software to build a prototype crowdsourcing platform that gave the Library the opportunity to test assumptions around the complexity and possibility of combining ML and volunteer contributions. This platform enabled hypothetical users to annotate Yellow Page directories from the 1940s-1960s through tasks such drawing boxes around advertisements and transcribing names, addresses, and phone numbers from business listings in order to extract structured business information that could help library users more easily find information relevant to their research.
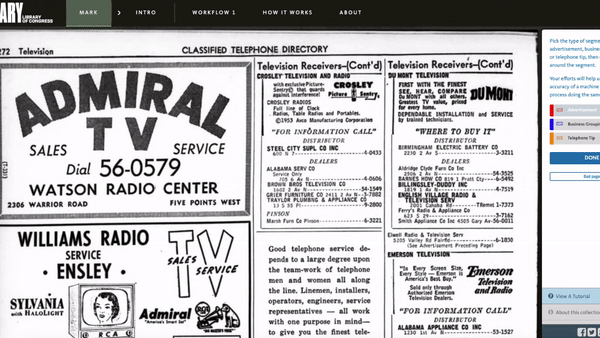
Because the enormous scale of Yellow Page directories in the United States would take humans decades to transcribe on their own, AVP experimented with training several machine learning methods on just a sample of human-extracted data so the machines could learn how to extract this information in a fraction of the time. Together, AVP and LC raised and explored many questions around ethical, technical, and practical challenges of such an approach.
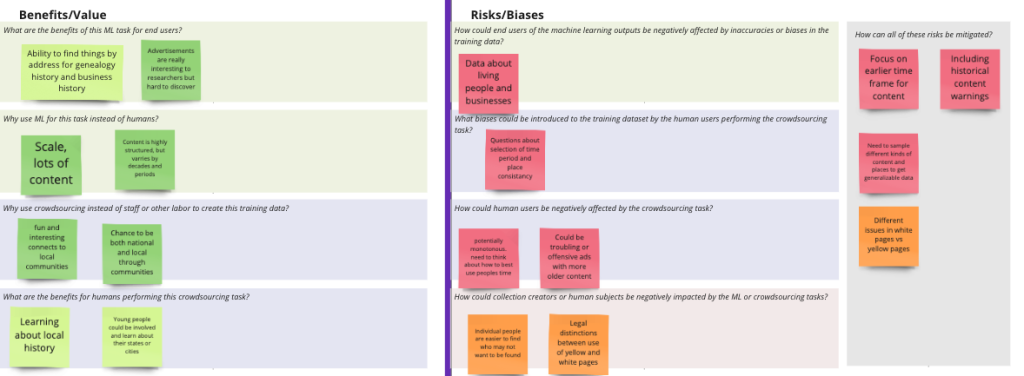
Remote user testing of the crowdsourcing prototype focused on gathering end-user perspectives on the use of ML in combination with crowdsourcing approaches. The volunteers offered overall positive responses, indicating that it is worthwhile to combine ML with volunteer contributions and that they would be willing to volunteer for human-in-the-loop projects. Even volunteers who explicitly expressed distrust of ML and AI approaches more generally indicated that knowing ML was involved in a crowdsourcing project would not deter them from volunteering, as long as the user experience of the platform was pleasing, and they found the tasks or content engaging.
Library of Congress also wanted to understand how the data generated through the human-in-the-loop processes might best be applied to enhance user-facing research experiences. To explore this question, AVP and LC worked together to create user personas and wireframes of a presentation interface based around defined use cases for the personas. AVP conducted user testing of the wireframes with volunteers engaged in various types of local history and genealogical research. User tests focused
on gathering feedback on how well the imagined design would support user interests and research goals. The tests surfaced useful feedback about overall design choices, research pathways, and features and functionality that researchers expected to see on a site built from human-in-the-loop processes.
After experimentation and user testing, AVP synthesized the experiences and results from this process to develop a reusable framework for any institution to apply in designing a human-in-the-loop project. The framework walks through four project stages—collection selection, project design, implementation, and presentation/sharing—and details the objectives, goals, and challenges of each stage, with recommendations on the humans to involve and tools that can help provide feedback in an iterative process. The goal of this framework is to help organizations understand how to carry out a human-in-the-loop project, how to resource potential projects with the right staff at the right time, and how to build risk assessment and mitigation into the project as an iterative practice to protect users.
The Impact
In the development of the experiment and framework, AVP and LC learned how some processes and technologies may be replicable across different human-in-the-loop projects and others will necessarily be custom to a particular collection or data generation goal, the knowledge of which will be useful to project resourcing in early planning stages. In engaging LC staff in many different roles throughout the experiment, the team learned what key staff and collaborations will be fruitful and even necessary at different stages of a human-in-the-loop project. The experiment demonstrated not only great potential for improvable machine learning processes in support of future human-in-the-loop projects at the Library of Congress, but also that human knowledge and contributions will continue to be critical to ensuring accurate machine learning results and continued care and respect for users.
Interested in learning more? Read the full project report and more on the LC Labs website.