Explore
Getting Started with AI for Digital Asset Management & Digital Collections
13 October 2023
Talk of artificial intelligence (AI), machine learning (ML), and large language models (LLMs) is everywhere these days. With the increasing availability and decreasing cost of high-performance AI technologies, you may be wondering how you could apply AI to your digital assets or digital collections to help enhance their discoverability and utility.
Maybe you work in a library and wonder whether AI could help catalog collections. Or you manage a large marketing DAM and wonder how AI could help tag your stock images for better discovery. Maybe you have started to dabble with AI tools, but aren’t sure how to evaluate their performance.
Or maybe you have no idea where to even begin.
In this post, we discuss what artificial intelligence can do for libraries, museums, archives, company DAMs, or any other organization with digital assets to manage, and how to assess and select tools that will meet your needs.
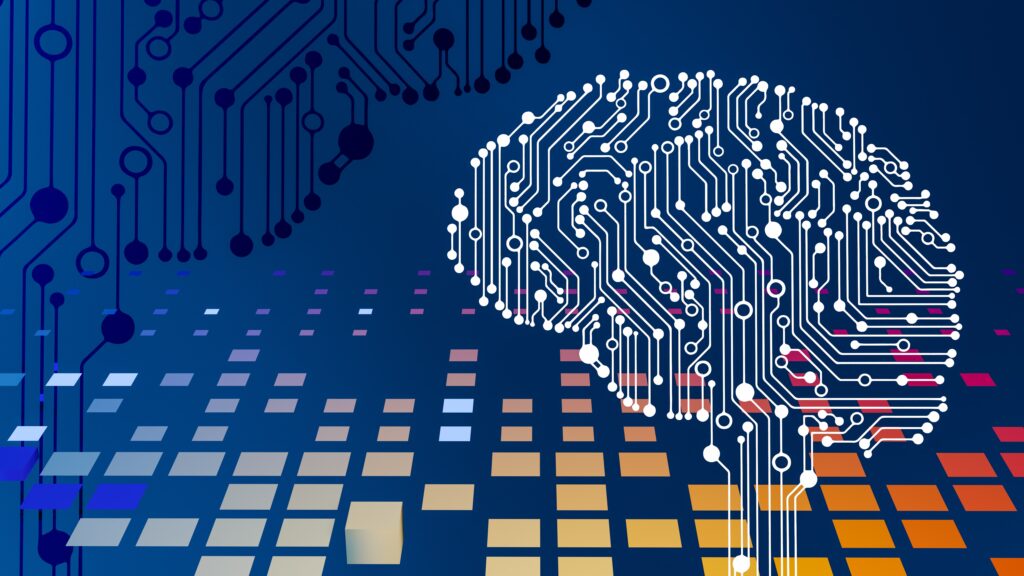
Examples of AI in Libraries and Digital Asset Management
Wherever you are in the process of learning about AI tools, you’re not alone. We’ve seen many organizations beginning to experiment with AI and machine learning to enrich their digital asset collections. We helped the Library of Congress explore ways of combining AI with crowdsourcing to extract structured data from the content of digitized historical documents. We also worked with Indiana University to develop an extensible platform for applying AI tools, like speech-to-text transcription, to audiovisual materials in order to improve discoverability.
What kinds of tasks can AI do with my digital assets?
Which AI methods work for digital assets or collections depends largely on the type of asset. Text-based, still image, audio, and video assets all have different techniques available to them. This section highlights the most popular machine learning-based methods for working with different types of digital material. This will help you determine which artificial intelligence tasks are relevant to your collections before diving deep into specific tools.
AI for processing text – Natural Language Processing
Most AI tools that work with text fall under the umbrella of Natural Language Processing (NLP). NLP encompasses many different tasks, including:
- Named-Entity Recognition (NER) – NER is the process of identifying significant categories of things (“entities”) named in text. Usually these categories include people, places, organizations, and dates, but might also include nationalities, monetary values, times, or other concepts. Libraries or digital asset management systems can use named-entity recognition to aid cataloging and search.
- Sentiment analysis – Sentiment analysis is the automatic determination of the emotional valence (“sentiment”) of text. For example, determining whether a product review is positive, negative, or neutral.
- Topic modeling – Topic modeling is a way of determining what general topic(s) the text is discussing. The primary topics are determined by clustering words related to the same subjects and observing their relative frequencies. Topic modeling can be used in DAM systems to determine tags for assets. It could also be used in library catalogs to determine subject headings.
- Machine translation – Machine translation is the automated translation of text from one language to another–think Google Translate!
- Language detection – Language detection is about determining what language or languages are present in a text.
AI for processing images and video – Computer Vision
Using AI for images and videos involves a subfield of artificial intelligence called Computer Vision. Many more tools are available for working with still images than with video. However, the methods used for images can often be adapted to work with video as well. AI tasks that are most useful for managing collections of digital image and video assets include:
- Image classification – Image classification applies labels to images based on their contents. For example, image classification tools will label a picture of a dog with “dog.”
- Object detection – Object detection goes one step further than image classification. It both locates and labels particular objects in an image. For example, a model trained to detect dogs could locate a dog in a photo full of other animals. Object detection is also sometimes referred to as image recognition.
- Face detection/face recognition – Face detection models can tell whether a human face is present in an image or not. Face recognition goes a step further and identifies whether the face is someone it knows.
- Optical Character Recognition (OCR) – OCR is the process of extracting machine-readable text from an image. Imagine the difference between having a Word document and a picture of a printed document–in the latter, you can’t copy/paste or edit the text. OCR turns pictures of text into digital text.
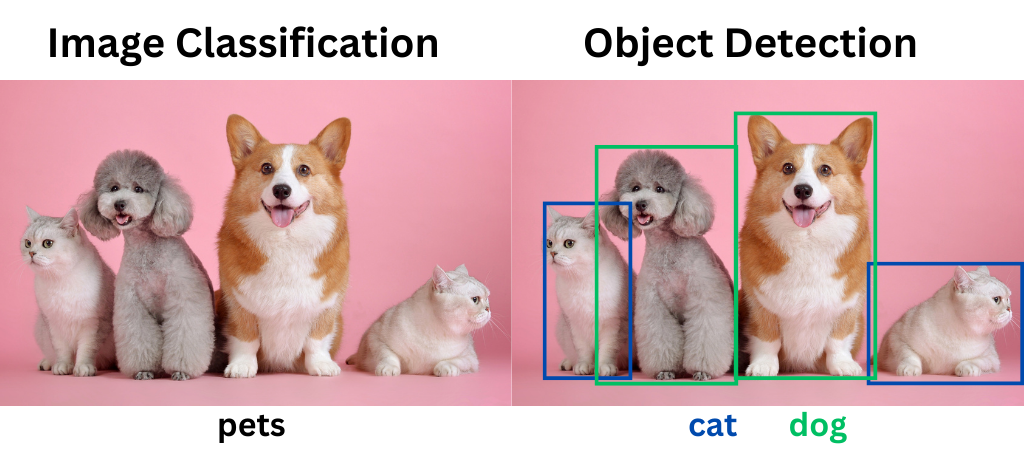
AI for processing audio – Machine Listening
AI tools for working with audio are much fewer and farther between. The time-based nature of audio, as opposed to more static images and text, makes working with audio a bit more difficult. But there are still methods available!
- Speech-to-text (STT) – Speech-to-text, also called automatic speech recognition, transcribes speech into text. STT is used in applications like automatic caption generation and dictation. Transcripts created with speech-to-text can be sent through text-based processing workflows (like sentiment analysis) for further enrichment.
- Music/speech detection – Speech, music, silence, applause, and other kinds of content detection can tell you which sounds occur at which timestamps in an audio clip.
- Speaker identification / diarization – Speaker identification or diarization is the process of identifying the unique speakers in a piece of audio. For example, in a clip of an interview, speaker diarization tools would identify the interviewer and the interviewee as speakers. It would also tell you where in the audio each speaks.
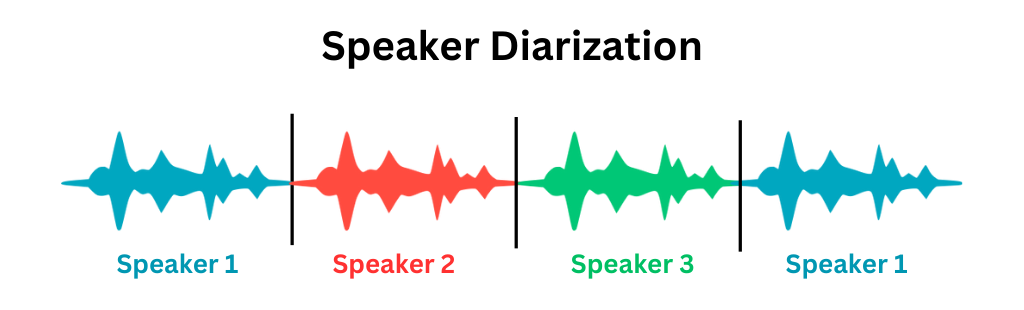
What is AI training, and do I need to do it?
Training is the process of “teaching” an algorithm how to perform its task.
Creating a trained machine learning model involves developing a set of training data, putting that data through a learning algorithm, and tweaking parameters until it produces desirable results.
You can think of training data as the “answer key” to a test you want the computer to take.
For example, if the task you want to perform is image classification–dividing images into different categories based on their contents–the training data will consist of images labeled with their appropriate category. During the training process, the computer examines that training data to determine what features are likely to be found in which categories, and subsequently uses that information to make guesses about the appropriate label for images it’s never seen before.
In addition to the labeled data given to the algorithm for learning, some data has to be held back to evaluate the performance of the model. This is sometimes called “ground truth” testing, which we’ll discuss more below.
Developing training and testing data is often the most time-consuming and labor-intensive part of working with machine learning tools. Getting accurate results often requires thousands of sample inputs, which may (depending on the starting state of your data) need to be manually processed by humans before they can be used for training.
Training AI tools sounds costly, is it always necessary?
Custom training may not be required in all cases. Many tools come with “pre-trained” models you can use. Before investing loads of resources into custom training, determine whether these out-of-the-box options meet your quality standards.
Keep in mind that all machine learning models are trained on some particular set of data.
The data used for training will impact which types of data the model is well-suited for—for example, a speech-to-text model trained on American English may struggle to accurately transcribe British English, and will be completely useless at transcribing French.
Researching the data used to train out-of-the-box models, and determining its similarity to your data can help set your expectations for the tool’s performance.
Choose the right AI tool for your use case
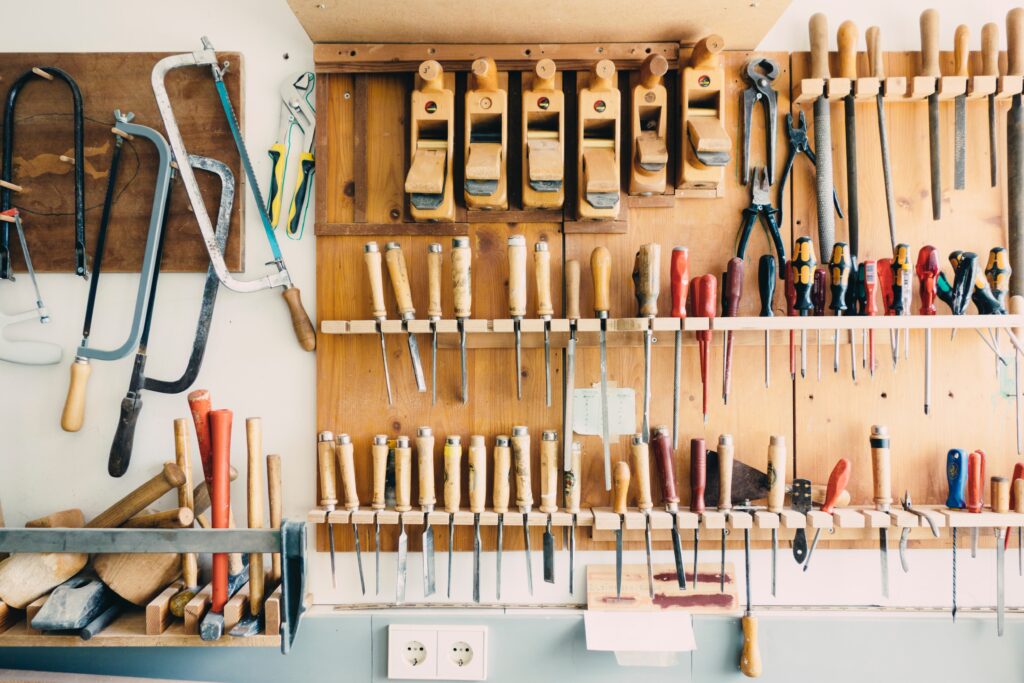
Before you embark on any AI project, it’s important to articulate the problem you want to solve and consider the users that this AI solution will serve. Clearly defining your purpose will help you assess the risks involved with the AI, help you measure the success of the tools you use, and help you determine the best way to present or make use of the results for your users in your access system.
All AI tools are trained on a limited set of content for a specific use case, which may or may not match your own. Even “general purpose” AI tools may not produce results at the right level of specificity for your purpose. Be cautious of accuracy benchmarks provided by AI services, especially if there is little information on the testing process.
The best way to determine if an AI tool will be a good fit for your use case is to test it yourself on your own digital collections.
How to evaluate AI tools
Ground truth testing is a standard method for testing AI tools. In ground truth testing, you create examples of the ideal AI output (ground truth) for samples of your content and check them against the actual output of the AI to measure the tool’s accuracy.
For instance, comparing the results of an object recognition tool against the list of objects you expect the tool to recognize in a sample of images in your digital asset management system can show you the strengths of the AI in correctly identifying objects in your assets (true positives) and its weaknesses in either not detecting objects it should have (false negatives) or misidentifying objects (false positives).
Common quantitative measures for ground truth testing include precision and recall, which can help you better calculate these risks of omission and misidentification. You can also examine these errors qualitatively to better understand the nature of the mistakes an AI tool might make with your content, so you can make informed decisions about what kind of quality control you may need to apply or if you want to use the tool at all.
Ground truth testing, however, can be costly to implement.
Creating ground truth samples is time-consuming, and the process of calculating comparison metrics requires specialized knowledge. It’s also important to keep in mind that ground truth can be subjective, depending on the type of tool—the results you’d expect to see may differ in granularity or terminology from the outputs the AI was trained to produce.
In the absence of ground truth, you can visually scan results for false positives and false negatives to get a sense of what kinds of errors an AI might make on your content and how they might impact your users.
Is it important that the AI finds all of the correct results? How dangerous are false positives to the user experience?
Seeing how AI results align with your answers to questions like these can help to quickly decide whether an AI tool is worth pursuing more seriously.
In addition to the quality of results, it is also important to consider other criteria when evaluating AI tools. What are the costs of the tool, both paid services and staff time needed to implement the tool and review or correct results? Will you need to train or fine-tune the AI to meet the needs of your use case? How will the AI integrate with your existing technical infrastructure?
To learn more about how you can evaluate AI tools for your digital assets with users in mind, check out AVP’s Human-Centered Evaluation Framework webinar, which includes a quick reference guide to these and many other questions to ask vendors or your implementation team.
When not to use artificial intelligence
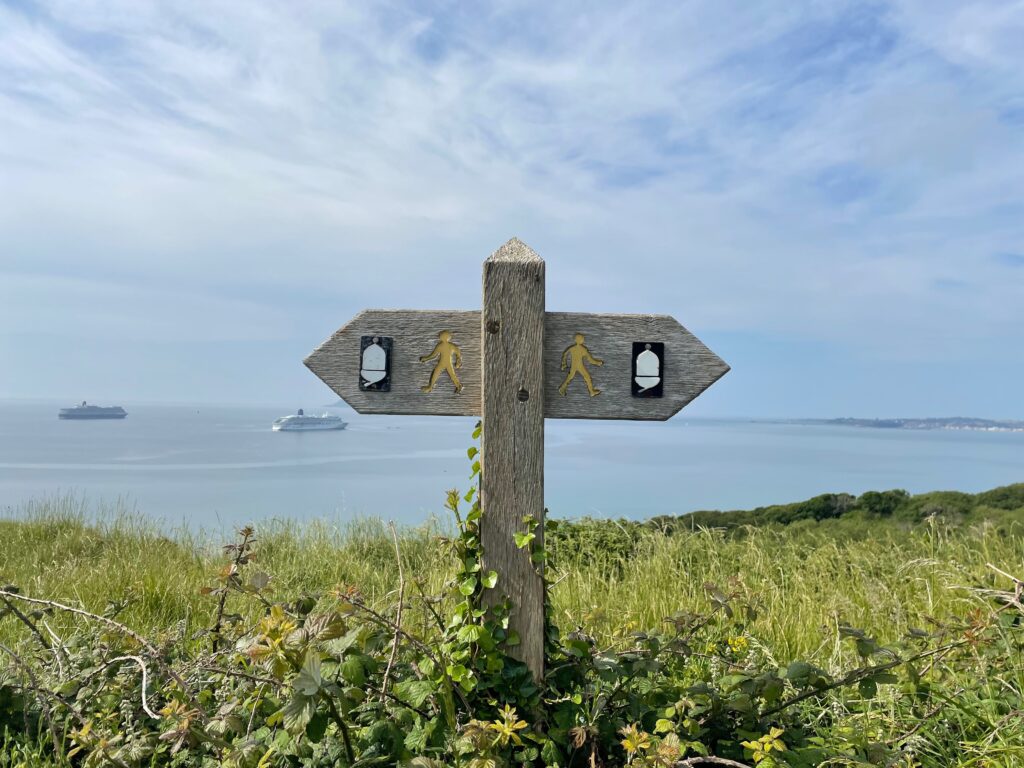
With all of the potential for error, how can you decide if AI is really worth it? Articulating your goals and expectations for AI at the start of your explorations can help you assess the value of the AI tools you test.
Do you want AI to replace the work of humans or to enhance it by adding value that humans cannot or do not have the time to do? What is your threshold for error? Will a hybrid human and AI process be more efficient or help relieve the tedium for human workers? What are the costs of integrating AI into your existing workflows and are they outweighed by the benefits the AI will bring?
If your ground truth tests show that commercial AI tools are not quite accurate enough to be worth the trouble, consider testing again with the same data in 6 months or a year to see if the tools have improved. It’s also important to consider that tools may change in a way that erodes accuracy for your use case. For that reason, it’s a good idea to regularly test commercial AI tools against your baseline ground truth test scores to ensure that AI outputs continue to meet your standards.
Now what?
The topics we’ve covered in this post are only the beginning! Now that you’ve upped your AI literacy and have a basic handle on how AI might be useful for enhancing your digital assets or collections, start putting these ideas into action.
Learn how AVP can help with your AI selection or evaluation project
Audiovisual Metadata Platform Pilot Development (AMPPD) Final Project Report
21 March 2022
This report documents the experience and findings of the Audiovisual Metadata Platform Pilot Development (AMPPD) project, which has worked to enable more efficient generation of metadata to support discovery and use of digitized and born-digital audio and moving image collections. The AMPPD project was carried out by partners Indiana University Libraries, AVP, University of Texas at Austin, and New York Public Library between 2018-2021.
Report Authors : Jon W. Dunn, Ying Feng, Juliet L. Hardesty, Brian Wheeler, Maria Whitaker, and Thomas Whittaker, Indiana University Libraries; Shawn Averkamp, Bertram Lyons, and Amy Rudersdorf, AVP; Tanya Clement and Liz Fischer, University of Texas at Austin Department of English. The authors wish to thank Rachael Kosinski and Patrick Sovereign for formatting and editing assistance.
Funding Acknowledgement: The work described in this report was made possible by a grant from the Andrew W. Mellon Foundation.
Read the entire report here.
PROBLEM STATEMENT
Libraries and archives hold massive collections of audiovisual recordings from a diverse range of timeframes, cultures, and contexts that are of great interest across many disciplines and communities.
In recent years, increased concern over the longevity of physical audiovisual formats due to issues of
media degradation and obsolescence, 2 combined with the decreasing cost of digital storage, have led institutions to embark on projects to digitize recordings for purposes of long-term preservation and improved access. Simultaneously, the growth of born-digital audiovisual content, which struggles with its own issues of stability and imminent obsolescence, has skyrocketed and continues to grow exponentially.
In 2010, the Council on Libraries and Information Resources (CLIR) and the Library of Congress reported in “The State of Recorded Sound Preservation in the United States: A National Legacy at Risk in the Digital Age” that the complexity of preserving and accessing physical audiovisual collections goes far beyond digital reformatting. This complexity, which includes factors such as the cost to digitize the originals and manage the digital surrogates, is evidenced by the fact that large audiovisual collections are not well represented in our national and international digital platforms. The relative paucity of audiovisual content in Europeana and the Digital Public Library of America is a testament to the difficulties that the GLAM (Galleries, Libraries, Archives, and Museums) community faces in creating access to their audiovisual collections. There has always been a desire for more audiovisual content in DPLA, even as staff members recognize the challenges and complexities this kind of content poses (massive storage requirements, lack of description, etc.). And, even though Europeana has made the collection of audiovisual content a focus of their work in recent years, as of February 2021, Europeana comprises 59% images and 38% text objects, but only 1% sound objects and 2% video objects. DPLA is composed of 25% images and 54% text, with only 0.3% sound objects, and 0.6% video objects.
Another reason, beyond cost, that audiovisual recordings are not widely accessible is the lack of sufficiently granular metadata to support identification, discovery, and use, or to support informed rights determination and access control and permissions decisions on the part of collections staff and users. Unlike textual materials—for which some degree of discovery may be provided through full-text indexing—without metadata detailing the content of the dynamic files, audiovisual materials cannot be located, used, and ultimately, understood.
Traditional approaches to metadata generation for audiovisual recordings rely almost entirely on manual description performed by experts—either by writing identifying information on a piece of physical media such as a tape cassette, typing bibliographic information into a database or spreadsheet, or creating collection- or series-level finding aids. The resource requirements and the lack of scalability to transfer even this limited information to a useful digital format that supports discovery presents an intractable problem. Lack of robust description stands in the way of access, ultimately resulting in the inability to derive full value from digitized and born-digital collections of audiovisual content, which in turn can lead to lack of interest, use, and potential loss of a collection entirely to obsolescence and media degradation.
Read the entire report here.
AVP’s Digital Asset Management for Museums & Cultural Heritage
21 January 2022
AVP is an information innovation firm.
We bring a cross-disciplinary team of experts to help organizations transform how they protect, manage, and use data and digital assets. AVP’s expert team has over 15 years of experience designing holistic, user-based solutions to protect and deliver cultural heritage content to key audiences. We work alongside you to create impactful digital asset management solutions tailored to your organization and empower you to grow and sustain them.
AVP Digital Asset Management Services Map for Museums & Cultural Heritage
Plan
- Digital Asset Management Strategy
- Roadmapping
- Resource Planning & Advocacy
Launch
- Implementation Planning
- System Configuration
- Digital Asset Security
- User Testing
Pilot
- UX Research & Design
- Machine Learning/AI
- MVP Development
Breakthrough Workshops
- Lightning Decision Jam
- Creating a Guiding Vision
- Data Selection & Prioritization
- Search, Browse, and Discovery
Build
- Custom Software Solutions
- Workflow Automation
Connect
- Data Migration
- System Integration
Key
- Plan
- Select
- Launch
- Build
- Pilot
- Connect
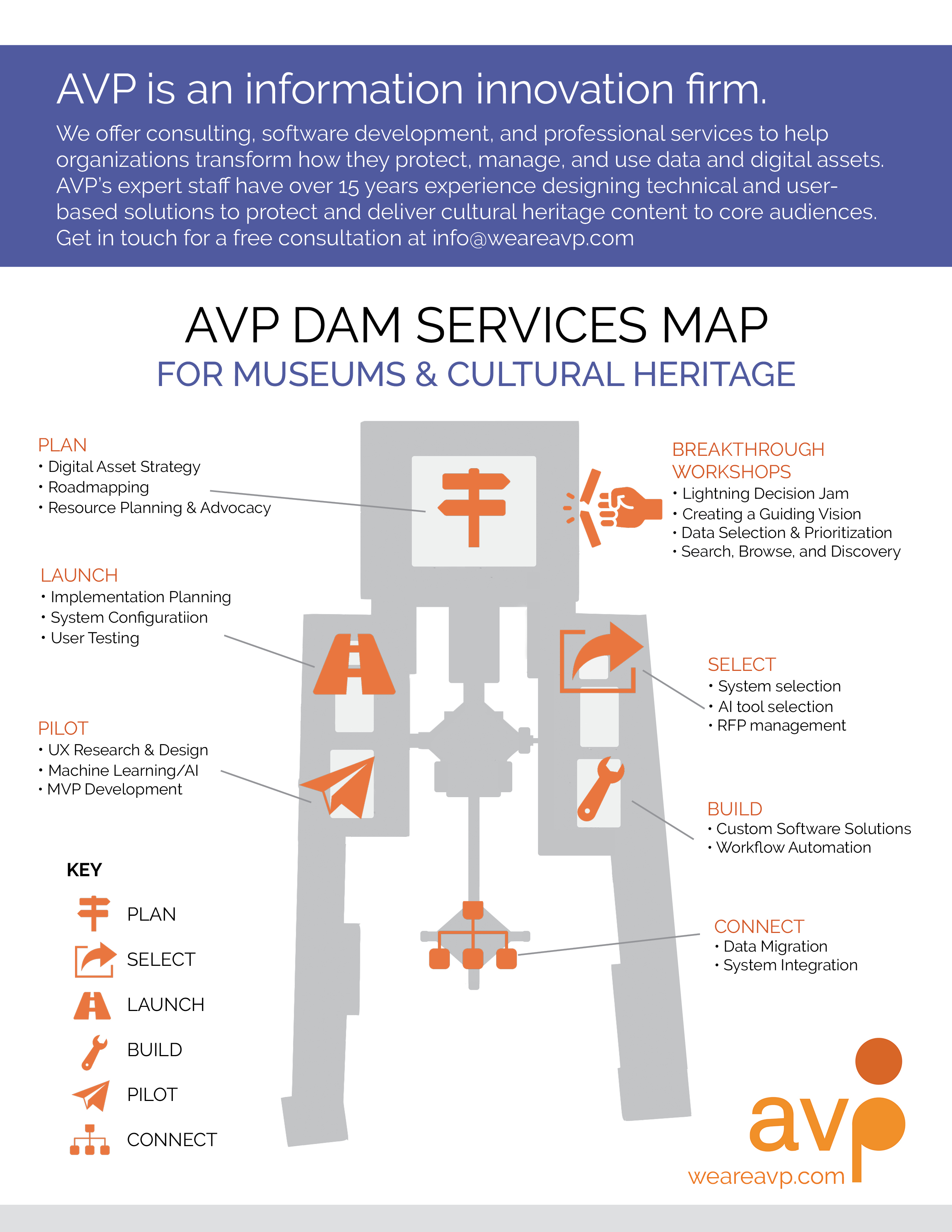
Who We Work With
We have been honored to partner with some amazing museums and cultural heritage organizations:
- Smithsonian Institution
- Museum of Modern Art (MoMA)
- Library of Congress
- National Museum of American History
- National Gallery of Art
- The Frick Collection
- John F. Kennedy Presidential Library Foundation (Library & Museum)
- United Nations
- United States Holocaust Memorial Museum
- Wisconsin Veterans Museum
- Rock and Roll Hall of Fame
- Country Music Hall of fame
- The Huntington
Each client had distinct goals and objectives and a unique mix of people, assets, budgets, systems, policies, and workflows. AVP’s cross-disciplinary team of digital asset management experts helped chart a path to success and deliver impactful results using a combination of the following services:
- AVPPlan to define a path toward successful outcomes through effective and sustainable asset management
- AVPSelect to narrow down which technologies best fit client requirements, use cases, and budget
- AVPActivate to launch, build, grow and expand an organization’s digital asset management program
- AVPExplore to prototype, test, and deploy different concepts to bring your ideas to life
Smithsonian Institution: Preserving the Nation’s Past, Preparing for the Future
AVP has had the pleasure of working with the Smithsonian Institution on a few occasions to deliver:
- A comprehensive look at the current state of preservation operations
- Analyzed risks and gaps
- Top challenges and corresponding recommendations
- Policy improvements to support digital preservation
Our deliverable included eight overarching phases to ensure the Smithsonian’s valuable digital content persists far into the future.
What Our Clients Are Saying
“They are highly knowledgeable, are experienced working with museums and cultural institutions, extremely professional and focused.”
National Museum Client
“What sets your team apart is the way you combine top subject matter expertise with great listening and communication skills.”
Public University Client
“I most appreciated the initiative, flexibility, as well as the creativity they were willing to provide during this process…I was most impressed by the high quality of customer service provided.”
Private University Client
“…People were pleasantly surprised about the clarity of the report and presentation, particularly on a topic that is historically dry and goes over people’s heads.”
National Museum Client
“Highly intelligent, professional approach to assessing our needs and very reliable timetable, budget adherence and super deliverables.”
National Museum Client
“They have a strong work ethic and are very responsive to customer needs.”
National Museum Client

Put Your Digital Assets to Work
Ready to put your digital assets to work?
AVP’s approach to digital asset management encompasses digital preservation, digital collection management, DAM, and MAM to maximize the value of these assets to the organization and its internal and external users.
Free Digital Preservation Webinar With AVP
8 December 2021
Free Digital Preservation workshop with AVP:
“Digital Preservation Maturity Assessment with AVP’s Digital Preservation Go!”
December 7, 2021 at 1 PM ET
Join AVP for a free webinar that will introduce you to our newest and lowest-cost digital preservation assessment service. During the webinar, Senior Consultant Amy Rudersdorf will present on the benefits of maturity assessments and how performing them can move your digital preservation program to the next level. Sign up here and learn more about DPGo! below.
New from AVP: AVP’s Best DAM Workshop Series
27 August 2021
Let AVP’s expert facilitators help transform your data or digital asset management program, in just a few hours. Our workshops have proven results and are designed to breakthrough tough challenges quickly. Read more about our workshop offerings below and reach out to us at [email protected] to learn more.
Sign Up For Free DAM Workshop With AVP 08/17/21 @ 1PM ET
23 June 2021
Join AVP for a free, interactive, one-hour workshop on August 17 (@ 1 pm ET) designed to help you improve your users’ digital asset management experience. During the workshop, AVP Director of Consulting Kara Van Malssen will lead participants through user-centered exercises that DAM program leaders can utilize to create a more useful search, browse, discovery, and usability experience. In this workshop, you will learn how to:
- Understand users’ mental model of assets so that they can be organized and presented in meaningful ways
- Identify common search and browse terminology so that assets can be made findable in ways that match users’ search behavior
- Identify what users want to know about assets so that they can be effectively used
These exercises have proven results and are the same we use when engaging with our clients in diverse industries and organizational sizes.
Click here to apply for the workshop.
This workshop will be (virtually) hands-on and interactive, so come prepared to participate. Space is limited and registration is not guaranteed but we will do our best to accommodate interest. Limit 2 people per organization, please register separately.
Feel free to check out other helpful AVP resources for digital asset management.
- Designing a User-driven DAM Experience, Part 1
- Getting to Success: A Scenario-driven Approach for Selecting, Implementing, and Deploying Digital Asset Management Systems
- AVP Tips to Manage Your DAM Expectations Video
- Change is hard. Rally around your vision.
We know what it takes to implement and launch a successful DAM. Our experts are always two steps ahead to ensure that surprises and oversights are eliminated, your DAM implementation meets your needs, your launch is smooth, and your users are delighted. We offer implementation packages or can create a tailored service based on your needs. Ready to talk about your project? Get in touch!
New AVP Report: Best Practices in Electronic Transfers
27 April 2021
Black Lives Matter: AVP Accountability Report #1
12 February 2021
In June of 2020 we published a statement titled Black Lives Matter: What We Believe and What We Will Do.
AVP Services for DLFforum & DigiPres
12 November 2020
AVP’s years of involvement in the digital preservation and libraries community has shaped the services we provide. With our deep understanding of needs and challenges, we have developed a mix of services, products, and resources for organizations of all sizes. Explore them below and reach out when you are ready to discuss a project.
Services
Digital Preservation Assessments and Audit Packages– Identify areas of need, prioritize, and plan for next steps
New Aviary Customer Support Packages
9 November 2020
We want to make sure you love the Aviary experience! We can help get you started, keep you supported, and elevate your use with the client support services below. Reach out for a conversation about customizing a package for your collections today.